A flying eye in the sky is ready to transform how we get groceries, receive medicine, and eventually how we commute to work. While many remain skeptical, experts predict that the use of drones, also referred to as unmanned aircraft systems (UAS), will increase at a 9.4% CAGR at the global level and reach $41.3 billion in revenue by 2026. Commercial rather than recreational drones are driving this growth, with enterprise drone solutions seeing the most significant increases.
The race is on to combine onboard artificial intelligence (AI), computer vision, and machine learning with drones’ exploratory and transportation abilities so people can receive anything from a pepperoni pizza to life-saving blood transfusions safely and quickly within cities, suburban, and rural areas.
The challenge is that as drone companies race to innovate, they are left with massive amounts of data to process and label. Like autonomous vehicles, there is no room for error in the models that help drones navigate, as faulty models can injure humans, animals, or property. High-quality data is absolutely essential to train machine learning and computer vision algorithms. Thus, image annotation is a critical step in preparing the massive amount of complex training data needed to train algorithms that drones use for autonomy in delivery and the movement of humans.
Drone technology is moving forward very quickly, and this guide will keep pace. We’ll regularly track and publish content about this exciting area and include new drone AI and computer vision applications to keep you looped into how drone delivery companies are enhancing their services. So be sure to bookmark this page and check back regularly for new information.
What we will cover in the guide: First, we’ll discuss the importance of adding AI and computer vision to your drone delivery operations if you plan to apply for the FAA’s Part 135 certification.
Then, we’ll explain the five main applications of AI that will be crucial as the drone delivery market emerges. The five applications are obstacle detection and collision avoidance, GPS-free navigation, contingency management and emergency landing, delivery drop, and safe landing.
Finally, we’ll cover the importance of collecting and labeling high-quality data to improve the safety and reliability of your drone and scale delivery operations quickly.
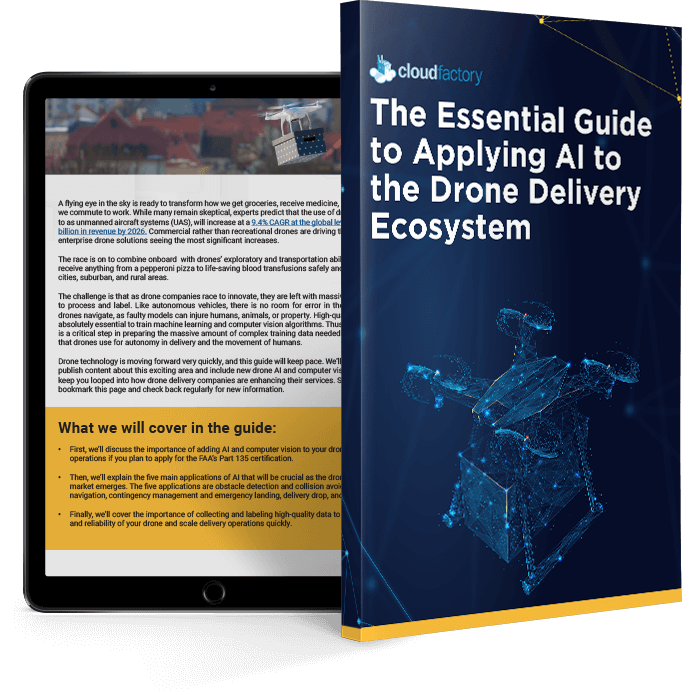
Read the full guide below, or download a PDF version of the guide you can reference later.
Table of Contents
Introduction:
Will this guide be helpful to me?
This guide will be helpful to you if you want to:
- Be prepared to apply for the FAA’s Part 135 certification, the only path for small drones to carry the property of another for compensation Beyond Visual Line of Sight (BVLOS).
- Meet the needs and expectations of customers in drone delivery services.
- Stay ahead of your competition by innovating your drone operations.
- Improve the performance of your drone through the use of AI, computer vision, and other automation techniques.
- Learn how increasing drone functionality will improve the quality and scale of your business.
- Deepen your knowledge about the importance of collecting, processing, and labeling high-quality data to improve the safety, predictability, and scalability of your drone delivery operation.
Background:
Regulatory Hurdles and The Reality of Drone Autonomy
The emerging drone delivery market must first overcome regulatory hurdles driven by existing aviation laws that focus on a pilot making decisions in the cockpit of an aircraft. To operate drone delivery at scale, drone operators need Beyond Visual Line of Sight (BVLOS) flight clearance and to go through the Part 135 certification process from the FAA. While the process of obtaining Part 135 certification is a lengthy task, AI and computer vision will address some of the hurdles, ultimately leading to safe and predictable drone deliveries.
Computer vision replaces the pilot’s eyes, assisting the drone to “see” various types of objects or scenery while flying in mid-air, allowing the drone to interpret, interact, and make decisions that a pilot would typically make during flight. In addition, a high-performance onboard image processor and a drone neural network can enable object detection, classification, and tracking while in flight.
And, just like autonomous vehicles, there is no room for error in the machine learning models that help drones navigate, as faulty models can injure humans, animals, or property. Thus, image annotation is a critical step in preparing the massive amount of complex training data needed to train algorithms that drones would use for autonomy in delivery.
The reality of drone autonomy
Many drones are already automated, following pre-programmed flight paths, for example. However, there is a large gap between automated and autonomous drones. While adding AI to drones can classify them as mostly autonomous, the reality is that most drones operate automatically, with varying levels of autonomy occurring during different phases of flight.
We created a framework that conceptualizes and operationally defines the levels of drone autonomy. The key is to understand these different levels and what they look like in practice within the drone industry. The levels of drone autonomy range from 0 to 5, with only one drone company, Exyn, reaching Level 4 status in April 2021. Learn more in our blog post, Breaking Down the Levels of Drone Autonomy.
What’s behind the push for autonomous drone delivery?
Access to life-saving medicine and vaccines
Zipline drones began delivering life-saving medical supplies throughout Rwanda in 2016, becoming the first national drone delivery program at scale globally. Since then, there has been a demand for drone delivery in America, especially in remote, denied environments where vehicle or foot traffic is impractical. The desire to improve the quality of life for the elderly and disabled is also driving demand, as drones will deliver medications right to their doorstep.
America started testing drones for medical deliveries in 2018 when Wake Med and Matternet joined forces to deliver simulated medical packages across the Wake Med Health system in North Carolina. Then, in response to COVID-19, UPS and Atrium Health Wake Forest Baptist and Zipline were granted emergency Part 135 certification from the FAA to use drones to deliver COVID-19 vaccines and testing materials to health centers in North Carolina.
Access to quicker household deliveries
Food deserts are areas where people have limited access to healthy and affordable food. This may be due to having a low income or having to travel farther to find healthy food options. Without access to healthful foods, people may be at higher risk of diet-related conditions like obesity, diabetes, and cardiovascular disease. Drone delivery will provide access to people living in these areas where groceries stores and fresh food are not available within a walkable distance.
In addition, people in all areas of the country are demanding same-day and quicker-than-ever food and retail deliveries, and drones are increasingly being tested at retail stores, restaurants, and e-commerce warehouses. Drone delivery at scale will be cheaper than delivery by ground.
Five Applications of AI in the Drone Delivery Ecosystem
Applying AI to drone delivery operations will play a big part in addressing the significant regulatory hurdles currently in place. AI can assist drones in perceiving their surroundings, detecting and avoiding objects and other aircraft, and providing analytical feedback in real-time. Eventually, AI will allow drones to operate pilot-free BVLOS flights within an unmanned traffic management (UTM) system based on a set of sensor inputs that help detect weather conditions, other manned aircraft, other drones, and obstacles and events on the ground.
The following five applications will be crucial as the drone delivery market emerges: obstacle detection and collision avoidance, GPS-free navigation, contingency management and emergency landing, delivery drop, and safe landing.
Here are more details about each of those applications:
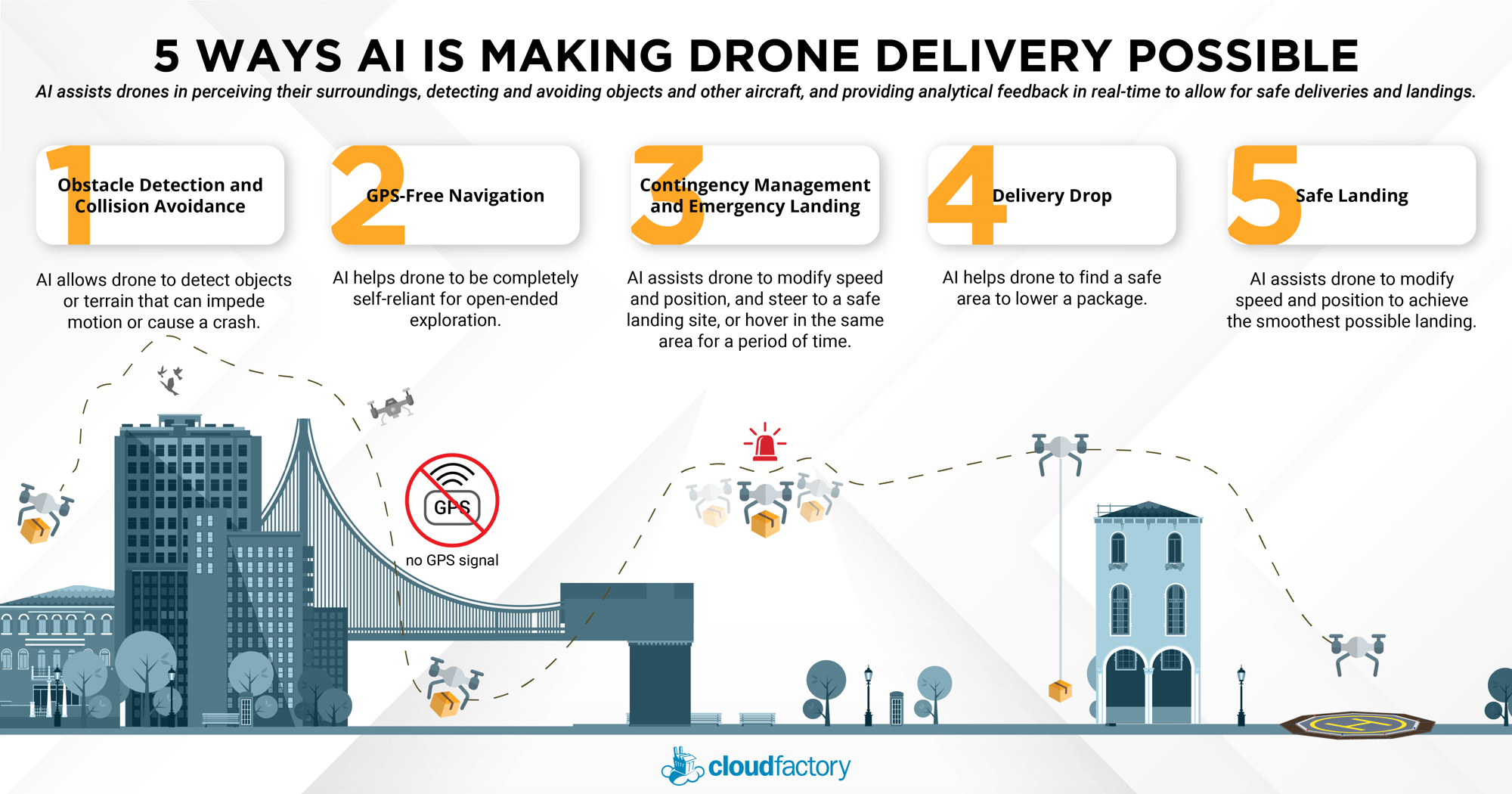
AI will assist drone delivery operators with five key applications: Obstacle detection and avoidance, GPS-free navigation, contingency management and emergency landings, delivery drop, and safe landings. These five applications of AI to drones are crucial as drone delivery companies seek Part 135 certification from the FAA.
1. Obstacle Detection and Collision Avoidance
In the expanding drone delivery market, obstacle detection and collision avoidance systems are in high demand. And as the FAA allows more and more drones to operate BVLOS, the need for drones to autonomously avoid collisions with objects and other aircraft is crucial because such drones operate out of the pilot’s visual range. AI will eventually support hundreds of drone operations within a UTM system, requiring the detection of other aircraft and the subsequent change in the flight path to quickly, accurately replicate the decisions a pilot would make looking out of the cockpit.
Application of AI: When applying AI to drones for obstacle detection and collision avoidance, computer vision software, sensors, thermal or infrared cameras, microphones (equipped with audio sensors), and optomechanical devices (optical mirrors and optical mounts) work together as a perception unit. Together, they collect a massive amount of data that train the drone to adjust for adverse environmental conditions, objects, and obstacles, both static and in motion.
Companies such as Iris Automation develop onboard computer vision software that allows drones to detect obstacles and avoid them, even at high speeds.
Amazon’s Prime Air drones are all equipped with sophisticated “detect and avoid” technology that captures the drone’s surroundings, allowing it to avoid obstacles or objects autonomously. The drones use computer vision and several sensors that constantly monitor the drone’s flight. These sensors are on all sides of the aircraft to spot things like an oncoming aircraft and other barriers.
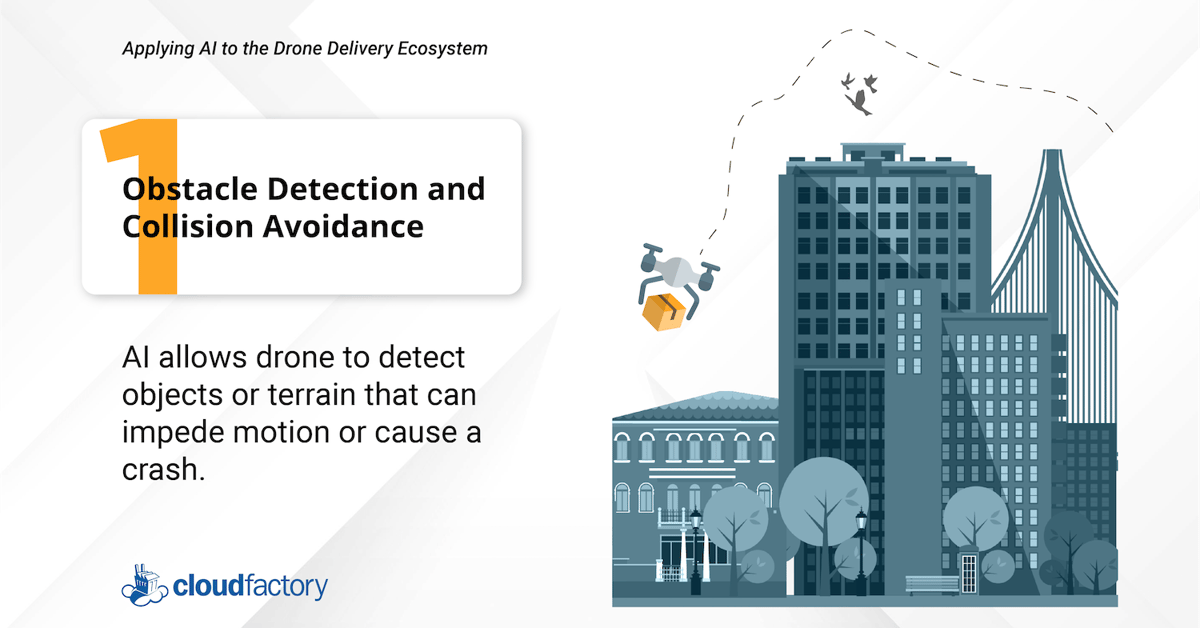
2. GPS-Free Navigation
Drone delivery will serve cities as well as suburban and rural communities. Cities present unique challenges such as urban canyons, which are areas within cities that restrict the view of the sky and reduce the number of satellites a drone can connect with. GPS-free, also called GPS-denied navigation, uses computer vision and AI to overcome those challenges.
Application of AI: When developing a drone’s mission, programmers use a combination of machine learning, deep neural networks, and reinforcement learning to train the drone to make a sequence of decisions to achieve a goal in an uncertain and potentially complex environment. Computer vision and sensors allow the drone to explore complex environments, including urban obstacles such as the areas around skyscrapers and bridges. AI will enable the drone to continue navigating its route by using visual sensors and an inertial measurement unit. Once the operator sets a flight plan, the drone can find the best way to get to its destination safely.
Exyn’s drones use GPS-free navigation to explore complex spaces. Their level 4 autonomous drones build a map of their surroundings while tracking their movement through that environment. As a result, drones are completely self-reliant for open-ended exploration and do not require human interaction during flight. This is a significant step up from level 3 autonomy, in which a human operator or pilot is required to be present and available to take control of the system at any time.
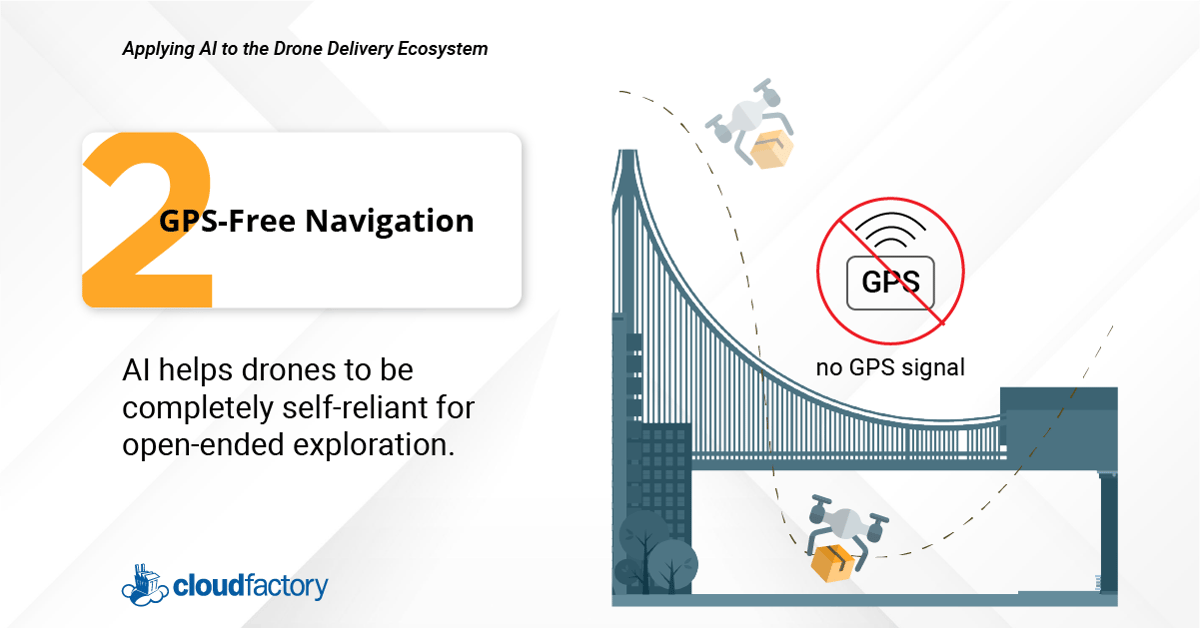
3. Contingency Management and Emergency Landing
The most in-demand and crucial application of AI lies in contingency management and emergency landings. Drone delivery operations will be impacted by a variety of factors, including weather, other aircraft, limited power supply, and component failures. AI and machine learning are helping to make emergency drone landings safer, especially for BVLOS flights. Even so, the reality is that drone delivery won’t happen on a large scale until drones can master the emergency landing.
Application of AI: In cases of emergencies or unpredictable circumstances, AI-enabled live data processing systems make predictions based on variables such as the drone’s current position, energy state, altitude, and wind speed, and then apply an algorithm to steer the drone to a safe landing site or hover in the same area until conditions change. Image sensors and AI-enabled object recognition software can help the drone find a safe landing place in the event of a forced landing due to system or flight failure or emergency conditions.
Zipline’s drones can make autonomous parachute landings in the case of an emergency, bringing the drone down to the ground in a safe, controlled descent. This video talks more about Zipline’s safety features.
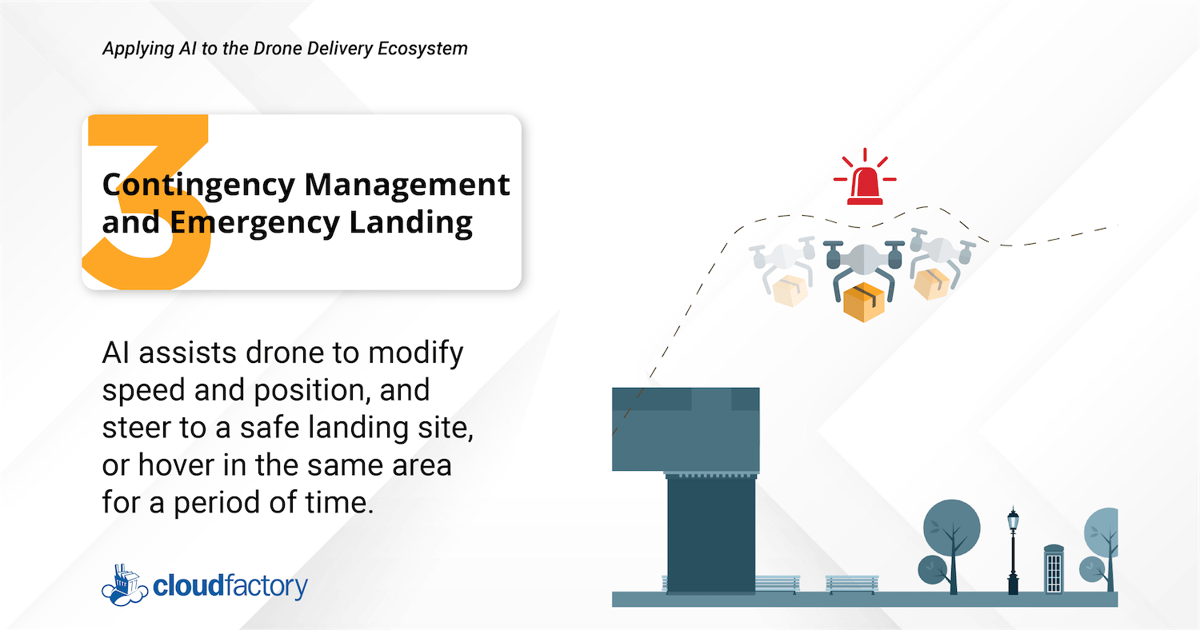
4. Delivery Drop
One critical phase of the drone delivery operation is delivering packages safely and in the correct locations. Many drone delivery companies lower packages via a parachute or string instead of landing the drone at the delivery site. Incorporating a delivery drop feature limits the recipient’s exposure to spinning propellers. It also keeps the drone from wasting time and battery power when maneuvering around obstacles like tree branches and power lines.
Application of AI: Computer vision combined with LiDAR and other sensors on the drone can measure how far the aircraft is from the ground and detect if anything obstructs its ability to safely lower the package to the ground.
Flytrex is a drone delivery service that will bring you anything from Chinese takeout to your morning coffee in minutes. Once a clear delivery space is identified using computer vision, the drone uses a wire release mechanism that gently releases food from about 80 feet, allowing for a quiet and safe delivery.
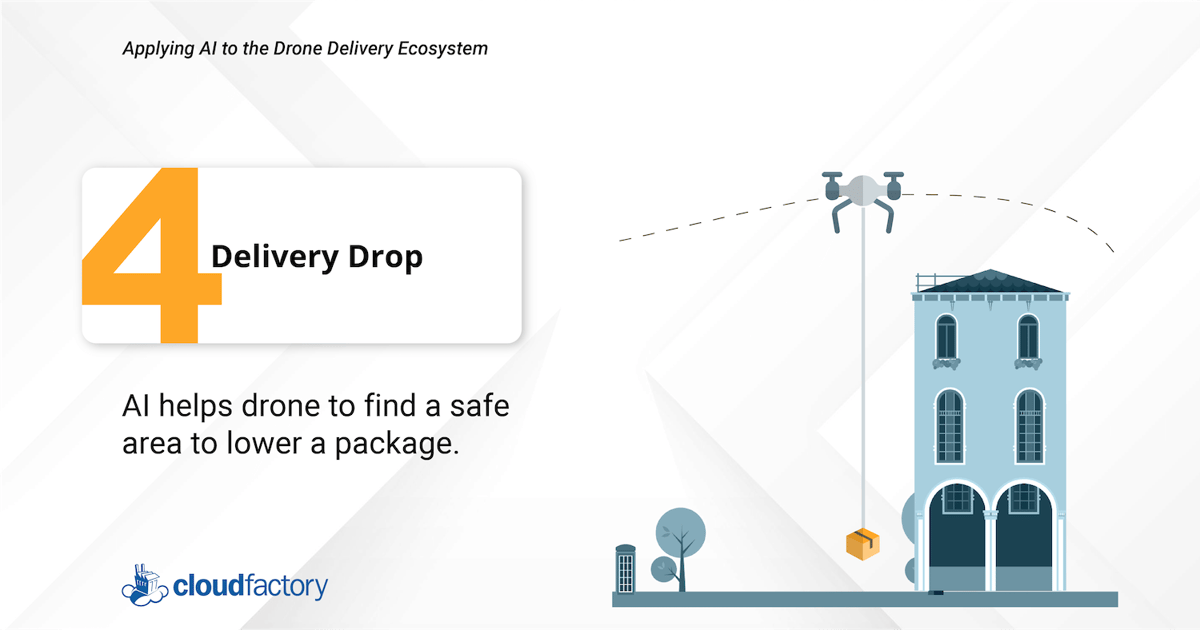
5. Safe Landing
There is a growing demand to apply AI and machine learning to routine drone landings, especially in urban environments where landing sites are small, and there is little room for error. Also, turbulence is common during the last moments of drone flight, sometimes causing irreparable damage to the drone. Unforeseen objects, people, animals, and debris can also cause unsafe landings. For drones to become mainstream, drones that must land to deliver consumer goods and life-saving medicines must do so safely, every single time.
Application of AI: AI-enabled sensors help track a drone’s position and speed and modify its landing trajectory and rotor speed accordingly to achieve the smoothest possible landing. Image sensors, AI-enabled object recognition, and drone flight computers combine to help land drones without the fear of overturning or damage.
Wing, the drone delivery company operated by Google’s parent company, Alphabet, regularly makes deliveries in Logan, Australia. Wing drones delivered over 10,000 coffees to residents from August 2020 through August 2021. When Wing drones return to the local distribution center, AI helps them safely and carefully land in small, designated spaces.
Matternet’s landing pod is an automated drone docking station that provides a safe place for a drone to land and swap its battery out. The docking station is a flower-like structure that protects deliveries from the elements and acts as a security system to guard medical payloads.
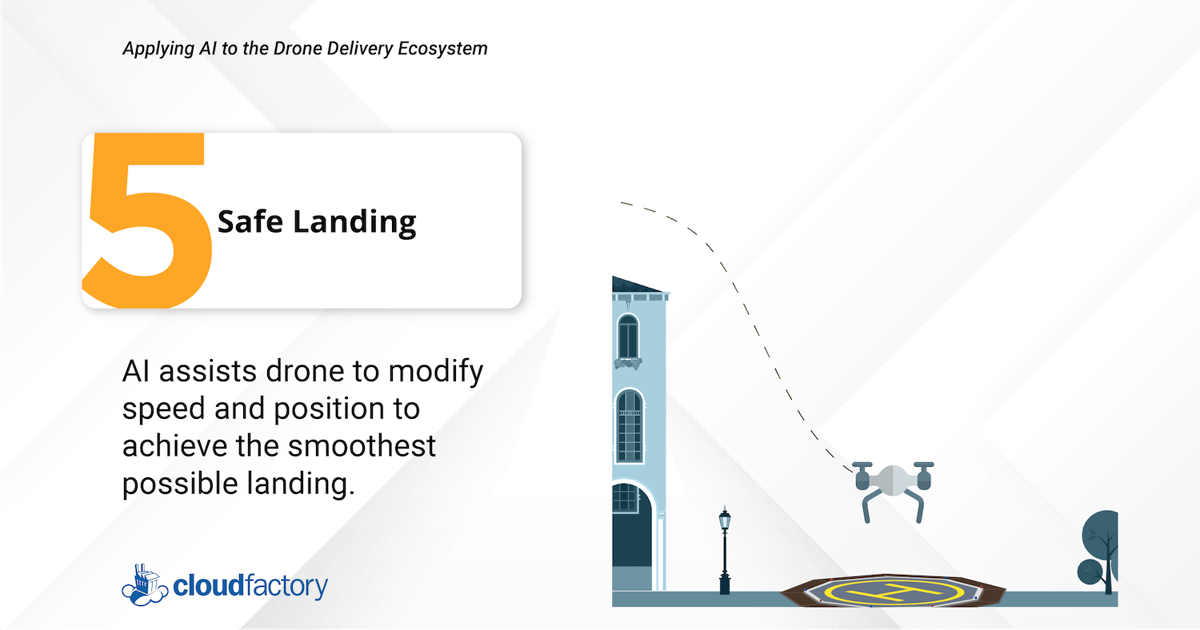
Collecting High-Quality Data in Drones
No element is more essential in the drone delivery ecosystem than high-quality training data, which is used to develop machine learning models that update and refine rules for a drone's mission as it operates. Fortunately, drones can collect large quantities of data from a multitude of sensors, scanners, and cameras during the course of normal operations.
For drone mission planning, quality training data generates thousands of gameplays where programmers either reward or penalize a model for the actions it performs. The quality of this data has profound implications for the model’s subsequent development, setting a powerful precedent for all future applications that use the same training data.
The Challenge: AI and Computer Vision Data Quality in Drones
If you’re part of a drone project team, the success of your project is directly tied to the volume of high-quality data you receive. Enormous amounts of data are required to keep your drones traveling from point A to point B, error, and accident-free.
Additionally, because data comes in from various sensors and cameras, labeling data to ensure a safe model is highly technical. It’s also incredibly daunting because of the resources and time involved in capturing, preparing, and labeling data. Sometimes, teams compromise on the quantity or quality of data – a choice that will undoubtedly lead to significant problems down the line.
Drone Data: Pre-processing, Live-processing, and Post-processing
There are only 24 hours in a day, and your drone team should consider the amount of time needed for pre-processing, live processing, and post-processing data. While it might not be glamorous work, it needs to get done, and details matter. That’s why finding a partner who can work through repetitive tasks with precision and communication is critical.
Pre-processing drone data
Pre-processing drone data can create exact replicas of real-world items such as machines, rooftops, or automobiles. When using drones for inspection purposes, a combination of 2-D images, lidar sensors, and photogrammetry can be applied to create 3-D renderings of the space, a process commonly referred to as digital twin creation.
Digital twins create a bridge between the physical and digital worlds. For example, in industries like drone delivery, digital twins can train one pilot to operate one drone and one pilot to operate many drones before moving on to a drone dispatch model where there is no pilot. Performing these types of experimental training programs in the digital world has lower risk at a lower cost than in the physical world.
Live-processing drone data
Live-processing enhances object detection, helping the drone to make game-time decisions such as avoiding other aircraft, flying around weather, avoiding structures, and the ability to make emergency landings. For example, a lake of oil is not safe, but a lake of water is. They may look similar if the drone uses simple sensors, but computer vision differentiates the lakes and adds infinite value to operations.
Post-processing drone data
Post-processing drone data can considerably improve drone delivery safety and efficiency. For example, sensor, image, and video data can indicate the level of weather distress a drone may experience during flight. Suppose data suggests that high winds during a particular season affect battery life or performance. In that case, operators can adjust the route or make other alterations to the flight plan. Post-data processing can also examine if pre-determined flight paths are optimal based on demand. If not, flight paths can be adjusted accordingly.
The Solution:
People, Process, and Technology
Drone companies can transform their data operations to produce quality training data with the right combination of people, processes, and technology. To do it requires seamless coordination between an expert human workforce, your machine learning project team, and your labeling tools.
People: Choosing the right people to annotate or label your AI data is one of the most important decisions you’ll make. Computer vision and sensor learning models are only as good as the data they are trained on, which means you will need people, often referred to as “humans in the loop,” to prepare and quality-check your image, video, and sensor annotations.
Process: Designing a computer vision model for drones is an iterative process. Data annotation evolves as you train, validate, and test your models. Along the way, you’ll learn from their outcomes, so you’ll need to prepare new datasets to improve your algorithm’s results.
Your data annotation team should be agile. That is, they must be able to incorporate changes in your annotation rules and data features. They also should be able to adjust their work as data volume, task complexity, and task duration change over time.
Your team of data annotators can provide valuable insights about data features - that is, the properties, characteristics, or classifications you want to analyze for patterns that will help train the machine to predict the outcome you’re targeting.
Technology: Data annotation tools are software solutions that can be cloud-based, on-premise, or containerized. You can use commercial tools to annotate pipelines of production-grade training data for machine learning or take a do-it-yourself approach and build your own tool. Alternatively, you can choose one of the many data annotation tools available via open source or freeware.
The challenge is deciding how to select the right tool for your computer vision project. Your first choice will be a critical one: build vs. buy. You’ll also have at least six important data annotation tool features to consider: dataset management, annotation methods, data quality control, workforce management, security, and integrated labeling capabilities.
Annotation Techniques for Drone Data
Drones collect large amounts of data while in flight. Annotation is the process of labeling or classifying data using text, annotation tools, or both, to show the data features you want your model to recognize on its own.
Annotation is a type of data labeling that is sometimes called tagging, transcribing, or processing. You also can annotate drone data from images, videos, sensors, lasers, and 3-D point clouds using LiDAR technology.
Annotation marks the features you want your machine learning system to recognize, and you can use the data to train your model using supervised learning. Once your model is deployed, you want it to be able to identify those features that have not been annotated and, as a result, make a decision or take some action.
Here are some of the common types of image annotations used in drone operations:
Bounding Box
Bounding boxes are used to draw a box around the target object, especially when objects are relatively symmetrical, such as vehicles, pedestrians, and road signs. It is also used when the object’s shape is of less interest or when occlusion is less of an issue. Bounding boxes can be 2-D or 3-D.
Tracking
Tracking is used to label and plot an object’s movement across multiple frames of video. Some image annotation tools have features that include interpolation, which allows an annotator to label one frame, then skip to a later frame, moving the annotation to the new position.
Polygon
A polygon is used to mark each of the highest points (vertices) of the target object and annotate its edges: These are used when objects have a more irregular shape, such as houses, areas of land, or vegetation.
Polyline
Polylines plot continuous lines made of one or more line segments: They are used when working with open shapes, such as road lane markers, sidewalks, or power lines.
3-D Cuboid
3-D laser scanners, RADAR sensors, and LiDAR sensors generate a point cloud where 3-D bounding boxes are used to annotate and/or measure many points on an external surface of an object.
2-D and 3-D Semantic Segmentation
2-D and 3-D laser scanners, RADAR sensors, and LiDAR sensors generate a point cloud where semantic segmentation is used to help train AI models by assigning each pixel in an image to a specific class of objects.
We’re on a mission.
At CloudFactory, our guiding mission is to create work for one million people in the developing world. We provide data analysts with training, leadership, and personal development opportunities, including community service projects. As data analysts gain experience, their confidence, work ethic, skills, and upward mobility increase, too. Our clients and their teams are an important part of our mission.
Are you ready to learn how to scale data annotation for autonomous drones and optimize your business operations with a flexible, professionally managed workforce? Find out how we can help you.
Contact Sales
Fill out this form to speak to our team about how CloudFactory can help you reach your goals.